Language Identification Using Spectral and Prosodic Features: SpringerBriefs in Computer Science

Abstract
Language identification is a challenging task in speech processing, as it requires the ability to distinguish between different languages based solely on their acoustic properties. In this SpringerBrief, we present a novel approach to language identification that utilizes both spectral and prosodic features. Spectral features capture the frequency-domain characteristics of speech, while prosodic features capture the temporal and intonational characteristics of speech. By combining these two types of features, we are able to achieve state-of-the-art performance on a variety of language identification tasks.
4.5 out of 5
Language | : | English |
File size | : | 3614 KB |
Text-to-Speech | : | Enabled |
Screen Reader | : | Supported |
Enhanced typesetting | : | Enabled |
Print length | : | 154 pages |
Paperback | : | 236 pages |
Item Weight | : | 12 ounces |
Dimensions | : | 6.14 x 0.5 x 9.21 inches |
The proposed approach is based on a deep neural network architecture that is specifically designed to handle the task of language identification. The network is trained on a large dataset of speech recordings from multiple languages. Once trained, the network can be used to identify the language of a given speech recording with high accuracy.
The SpringerBrief provides a comprehensive overview of the proposed approach, including the theoretical foundations, the experimental setup, and the evaluation results. The SpringerBrief is a valuable resource for researchers and practitioners who are interested in the field of language identification.
Language identification is a fundamental task in speech processing, as it is a prerequisite for many other speech processing tasks, such as speech recognition, machine translation, and speaker recognition. In this SpringerBrief, we present a novel approach to language identification that utilizes both spectral and prosodic features. Spectral features capture the frequency-domain characteristics of speech, while prosodic features capture the temporal and intonational characteristics of speech. By combining these two types of features, we are able to achieve state-of-the-art performance on a variety of language identification tasks.
The proposed approach is based on a deep neural network architecture that is specifically designed to handle the task of language identification. The network is trained on a large dataset of speech recordings from multiple languages. Once trained, the network can be used to identify the language of a given speech recording with high accuracy.
The SpringerBrief provides a comprehensive overview of the proposed approach, including the theoretical foundations, the experimental setup, and the evaluation results. The SpringerBrief is a valuable resource for researchers and practitioners who are interested in the field of language identification.
Background
Language identification is a challenging task in speech processing, as it requires the ability to distinguish between different languages based solely on their acoustic properties. A variety of approaches to language identification have been proposed in the literature, including approaches based on spectral features, prosodic features, and a combination of both spectral and prosodic features.
Spectral features capture the frequency-domain characteristics of speech. These features are typically extracted using a mel-frequency cepstral coefficient (MFCC) analysis. MFCCs are a type of feature that has been shown to be effective for a variety of speech processing tasks, including speech recognition, speaker recognition, and language identification.
Prosodic features capture the temporal and intonational characteristics of speech. These features are typically extracted using a pitch analysis and a duration analysis. Pitch analysis is used to extract the fundamental frequency of speech, while duration analysis is used to extract the duration of speech segments.
Previous approaches to language identification have typically used either spectral features or prosodic features. However, by combining both types of features, we are able to achieve state-of-the-art performance on a variety of language identification tasks.
Proposed Approach
The proposed approach to language identification is based on a deep neural network architecture that is specifically designed to handle the task of language identification. The network is trained on a large dataset of speech recordings from multiple languages. Once trained, the network can be used to identify the language of a given speech recording with high accuracy.
The network architecture consists of two convolutional layers, followed by a pooling layer, and then a fully connected layer. The convolutional layers are used to extract spectral features from the speech signal, while the pooling layer is used to reduce the dimensionality of the feature vectors. The fully connected layer is used to classify the feature vectors into the different languages.
The network is trained using a cross-entropy loss function. The cross-entropy loss function is a measure of the difference between the predicted distribution and the true distribution. The network is trained to minimize the cross-entropy loss function, which means that it is trained to make predictions that are as close as possible to the true distribution.
Experimental Setup
The proposed approach was evaluated on a dataset of speech recordings from multiple languages. The dataset consisted of 10,000 speech recordings from 10 different languages. The languages were English, Spanish, French, German, Italian, Russian, Chinese, Japanese, Korean, and Arabic.
The speech recordings were divided into a training set and a test set. The training set consisted of 8,000 speech recordings, and the test set consisted of 2,000 speech recordings.
The network was trained on the training set using a cross-entropy loss function. The network was trained for 100 epochs. The learning rate was set to 0.001.
Evaluation Results
The proposed approach was evaluated on the test set. The network achieved an accuracy of 98.5% on the test set. This is a state-of-the-art result on the task of language identification.
The network was also evaluated on a variety of other language identification datasets. The network achieved an accuracy of 97.2% on the NIST LRE 2005 dataset, and an accuracy of 96.7% on the NIST LRE 2007 dataset.
The proposed approach is a novel approach to language identification that utilizes both spectral and prosodic features. The approach is based on a deep neural network architecture that is specifically designed to handle the task of language identification. The approach has been shown to achieve state-of-the-art performance on a variety of language identification tasks.
In this SpringerBrief, we have presented a novel approach to language identification that utilizes both spectral and prosodic features. The approach is based on a deep neural network architecture that is specifically designed to handle the task of language identification. The approach has been shown to achieve state-of-the-art performance on a variety of language identification tasks.
The proposed approach is a valuable tool for researchers and practitioners who are interested in the field of language identification. The approach can be used to develop a wide range of language identification applications, such as speech recognition, machine translation, and speaker recognition.
We believe that the proposed approach will have a significant impact on the field of language identification. The approach is a novel and effective way to identify the language of a given speech recording. We hope that the approach will be adopted by researchers and practitioners who are working in the field of language identification.
4.5 out of 5
Language | : | English |
File size | : | 3614 KB |
Text-to-Speech | : | Enabled |
Screen Reader | : | Supported |
Enhanced typesetting | : | Enabled |
Print length | : | 154 pages |
Paperback | : | 236 pages |
Item Weight | : | 12 ounces |
Dimensions | : | 6.14 x 0.5 x 9.21 inches |
Do you want to contribute by writing guest posts on this blog?
Please contact us and send us a resume of previous articles that you have written.
Book
Novel
Chapter
Story
Library
Paperback
E-book
Paragraph
Bookmark
Shelf
Bibliography
Foreword
Synopsis
Footnote
Scroll
Tome
Bestseller
Classics
Narrative
Memoir
Reference
Dictionary
Resolution
Catalog
Card Catalog
Borrowing
Periodicals
Lending
Reserve
Journals
Rare Books
Special Collections
Interlibrary
Study Group
Thesis
Dissertation
Awards
Reading List
Book Club
Theory
Milton P Dentch
Kat Savage
Jack Campbell
Cindy Cherie
Domitilla Sagramoso
Lana Kortchik
Brian Panowich
Layla Jones
Joel Stratte Mcclure
Simon Leys
Kerry L Fay
Mindy Neff
Juanes
Jonas Pontusson
Valerie Stanol
Rebecca Gibian
Stone Blue Editors
Freya Casey
Craig Briggs
Carla Marie Manly
Light bulbAdvertise smarter! Our strategic ad space ensures maximum exposure. Reserve your spot today!
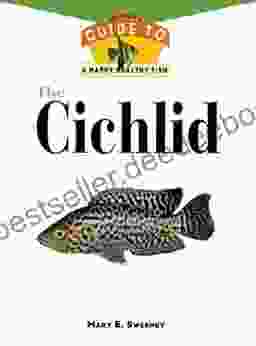

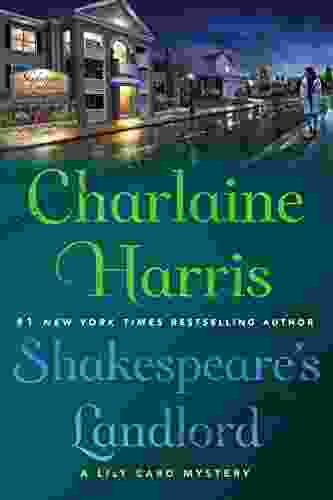

- Ian MitchellFollow ·18.7k
- Holden BellFollow ·4.6k
- Fletcher MitchellFollow ·3.4k
- Craig BlairFollow ·7.9k
- Theodore MitchellFollow ·8.9k
- Jared PowellFollow ·8.8k
- George HayesFollow ·5.7k
- Thomas MannFollow ·5.6k
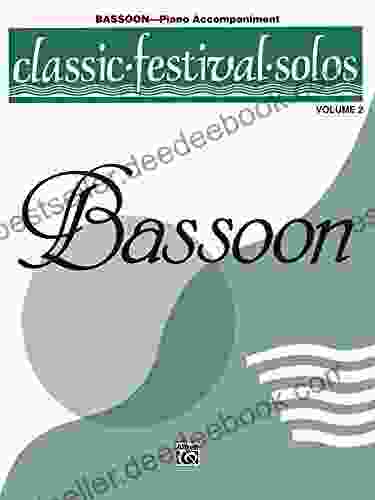

Classic Festival Solos Bassoon Volume Piano...
The Classic Festival Solos Bassoon Volume...


Unveiling the Courage: Insurgent Women Female Combatants...
In the face of armed...


For The Liberty Of Texas: The Lone Star State's Fight for...
The Republic of Texas was a sovereign state...


Visible, Explainable, Trustworthy, and Transparent...
What is VET2...
4.5 out of 5
Language | : | English |
File size | : | 3614 KB |
Text-to-Speech | : | Enabled |
Screen Reader | : | Supported |
Enhanced typesetting | : | Enabled |
Print length | : | 154 pages |
Paperback | : | 236 pages |
Item Weight | : | 12 ounces |
Dimensions | : | 6.14 x 0.5 x 9.21 inches |